Scientific Report 1136Permalink
This report is also available as a PDF document .
AbstractPermalink
The purpose of GNSS Loan 1136 was to support the deployment of an Unmanned Aerial Vehicle (UAV) collecting LiDAR data and digital aerial photographs (DAP) over remote tropical forests in Gabon, and Peru. The purpose of these field campaigns was to obtain measurements of forest recovery after controlled logging. The data were collected as part of a European Research Council (ERC) funded project titled FODEX (Tropical Forest Degradation Experiment), the aim of which is to use these data to improve our ability to map and monitor changes in forest structure and carbon stocks following human disturbance using satellite data. The pre-logging data in Peru was collected under Loan 1109, with the pre-logging data in Gabon, and post-logging data collected under Loan 1124.
BackgroundPermalink
The FODEX (Forest Degradation Experiment) is a 5-year experiment (July 2018 December 2023) that aims to shed new light on the status of the world’s tropical forests and how they are changing in response to human activities. The basis of the project is a twin large-scale field manipulation experiment with four one-ha forest inventory plots located within active logging concessions in the Peru and Gabon (Figure 1) (McNicol et al., 2021). Within each plot, the project collected Terrestrial Laser Scanning (TLS) data alongside traditional forest inventory measurements before and after controlled logging meaning the actual change in forest structure / aboveground tree volume and biomass can be used to train and test models of change (Aquino et al., 2022; Carstairs et al., 2022b, 2022a) (Figure 2). These ground data will be compared to LiDAR data collected via a 3.3m wingspan UAV (Delair DT26X UAV equipped with a Riegl miniVUX) and a 24 MP camera (Davenport et al., 2020; McNicol et al., 2021). The UAV is capable of collecting high-resolution (cm level) data on tree structure and health over large areas, information that is unobtainable using ground and/or satellite based platforms. These data have been collected several times over each concession and used to scale the ground data to give thousands of hectares of biomass change data.
In order to properly generate, and then combine these multi-scale datasets across multiple time-points, accurate and precise information on the location of the field plots and the flight trajectory of both of our UAVs is required. To that end, we requested the loan of two Leica GNSS Systems; one to act as Base Station for the purpose of correcting both the UAV trajectory, and the data from the second Rover system, which was used to collect additional ground control and reference points to further refine and correct LiDAR derived point clouds.
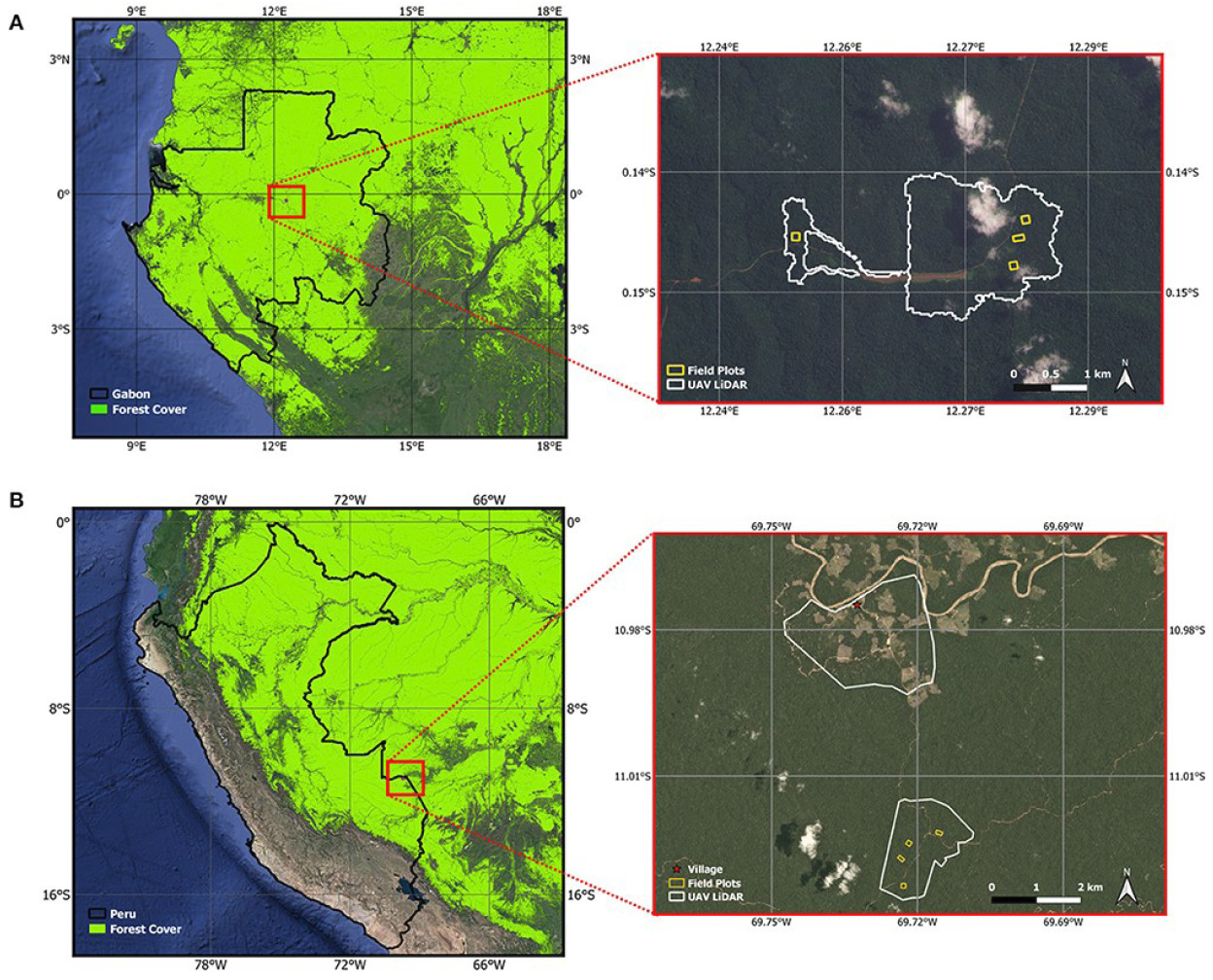
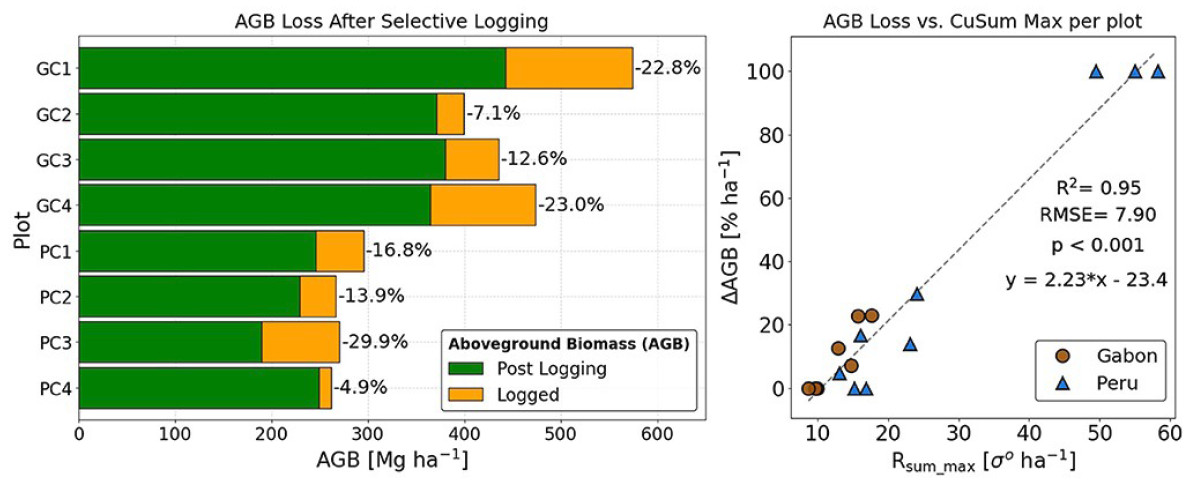
Survey ProcedurePermalink
The UAV with the LiDAR and RGB camera is equipped with a GNSS receiver capable of Post Processing Kinematic (PPK) correction, however, the nearest reference stations are several hundred kilometres from the field sites. The solution was to establish a temporary reference station at each field site, which can then be used as basis for a PPK correction of the UAV flight trajectory, and ground control points (GCPs) which will allow additional adjustments and validation (Figure 1).
As with our previous data collection campaigns, the Leica GS10 base receiver was deployed in a secure, but open area at each site (within 10 km of target area), and initially left for 18 24 hours to obtain enough data for an accurate and precise estimate of its position. A physical marker (typically metal re-bar inserted in ground) was used as a reference point to position the receiver. This process was repeated twice over the course of each field campaign (Table 1 and Table 2) to account for any uncertainty on the position, and ensure the position of the receiver remained static. The receiver runs in-sync (1 measurement/s) with the UAV and was set to record data for an hour before and after each day’s missions to allow PPK correction.
Prior to the commencement of the UAV missions a minimum of three (GCPs) - square targets 1-2 m2 in size composed of alternating black and white material arranged in a checkerboard pattern were placed across the road/ track network to allow further correction of the flight trajectory and help support co-registration during the processing of each mission (Figure 3). These were geo-located using the GS12 rover receiver, which was left a minimum of 3 - 5 minutes, but normally up 10 minutes over each target, or until the DOP values reached a plateau. As with the base station, repeat measurements are made over a subset of these GCPs/ marker points account for measurement error in the initial collection.
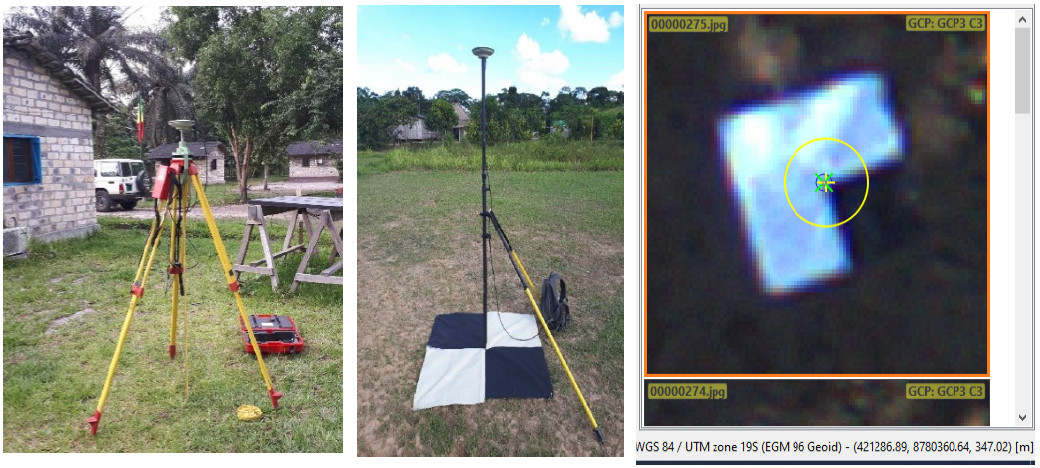
Processing and modellingPermalink
The GS10 base station and GS12 rover GNSS data were processed using the Leica Infinity software. The GS10 data were corrected using the Trimble CenterPoint RTX Post-Processing Service (Table 1 and Table 2). The rover receiver data were then tied to the base station in Infinity and the results exported in their local UTM coordinates. The flight trajectories of the LiDAR-RGB UAV were reconstructed using the GNSS/ IMU measurements and then adjusted using the differentially corrected base station data within the Applanix POSPac Software. The corrected flight paths and laser data were combined using the RIEGL software package, RiPROCESS to generate the initial laser 3D point cloud. Residual errors in the flight trajectory, e.g. due to discrepancies in GPS tracking and elevation, were identified and corrected in the LiDAR data using the small buildings for reference to further adjust the relative position and orientation (i.e. line-up) of individual laser scans. The trajectories were then further refined using the corrected GCPs (Figure 4) to produce the final LiDAR point cloud.
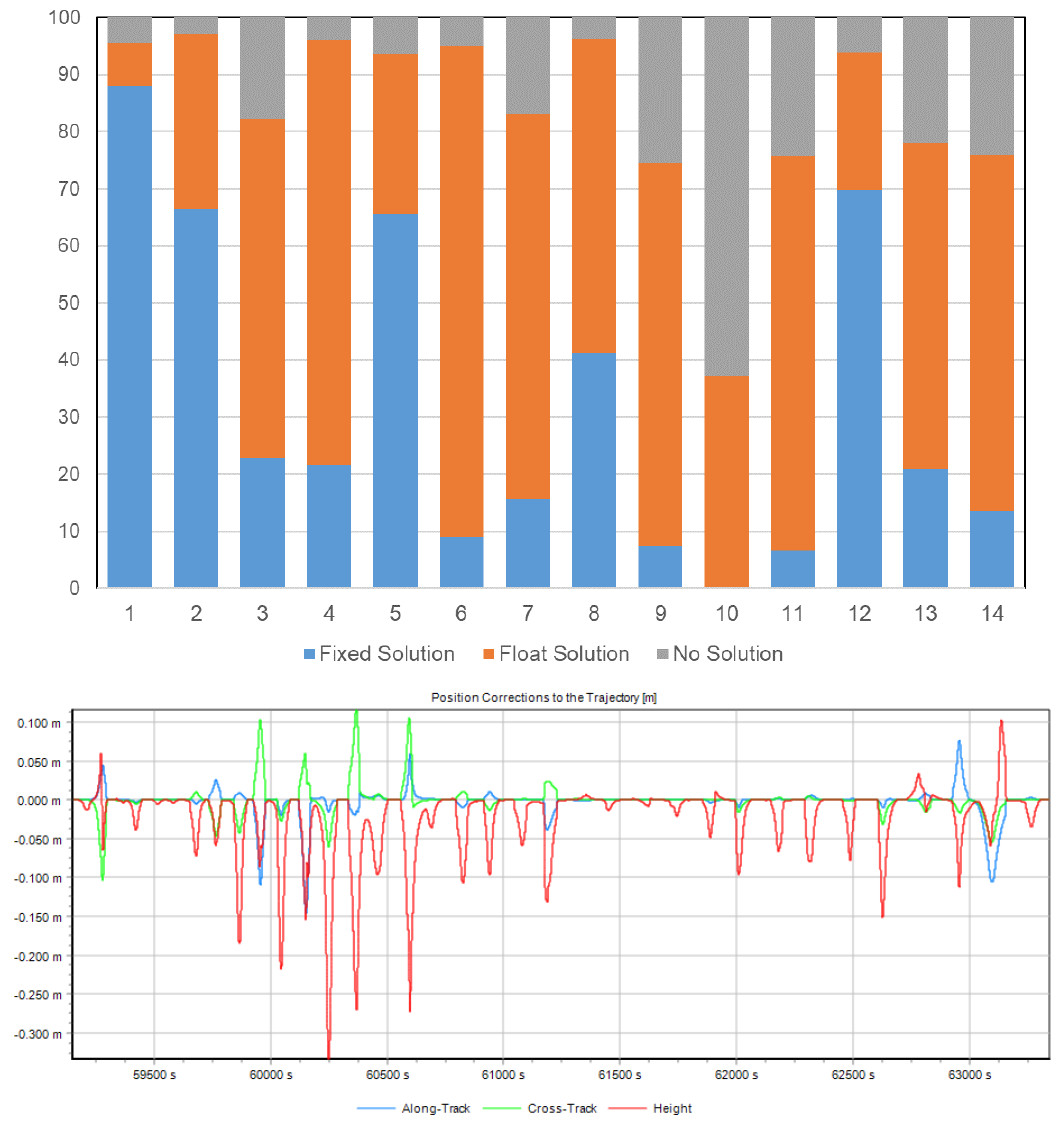
GNSS Data QualityPermalink
Overall, the PPK correction resulted in a relatively small (< 0.5 m), yet important adjustment to the UAV flight trajectories. However, in sharp contrast to our previous campaigns, we found that for nine of the fourteen missions conducted, a floating-point, or no solution was achieved for the UAVs positions across the majority of these flights. A visual analysis of the data showed that for these missions there were multiple cycle slips in the L2 data received by the UAV, the reasons for which are not entirely clear, and may reflect a combination of problems. One possible explanation is a hardware issue with the receiver; however, a caveat is that a fixed-point solution was achieved for the majority of the other four missions. Another potential cause may be due to how the missions themselves were conducted, including the length of time the drone spent in its default holding pattern after launch, and the stability of the drone when in flight. The standard operating procedure is to monitor the drone for 2 3 minutes at the start of each mission to check all systems are operating smoothly, and to allow the GNSS receiver to relocate all of the available satellites after the rapid launch. It is possible that as our experience of operating the UAV has increased the time spent checking the key functions of the drone has sped up accordingly, giving the GNSS receiver less time to locate all available satellites.
Whatever the root cause, the GCPs were a valuable addition to the data processing as they allowed additional adjustments to be made to the final point cloud. As this was the third time the sites have been covered, we also used the previous LiDAR data to generate an additional 30 40 manual reference points at each site. These were including mainly to correct the height data (z-coordinate) using reliable measurements of the ground surface collected along the road and track networks, with the corners of buildings / solar panels also used to help refine the x,y coordinates. As with our previous measurement campaigns, we achieved a fixed solution for the majority of the field-measured GCPs in both Peru and Gabon, with the absolute distance between repeatedly measured GCPs (x,y,z) averaging 3.6 cm [min = 0.019 cm; max = 8 cm] (Tables 1 & 2). One of the GCPs in Gabon was excluded as it appears to have been moved from its original position, with another three GCPs lost across the two study sites. This has been a recurring problem over the course of the project with wind, animals and humans all playing a part. The placement of the GCPs has been a challenge given the vegetation density, and the lack of open space, which means these necessarily have to be placed close to or along the road and track networks.
Interpretation and Preliminary findingsPermalink
The data collected under this Loan has not yet resulted in any publications, however, there are currently two manuscripts in preparation that use the UAV-LiDAR data collected in Gabon. There have been another two manuscripts published that use the data collected under Loans 1109 and 1124. Both of these manuscripts use freely available Sentinel-1 data to map areas of tree cover loss across Gabon (Carstairs et al., 2022b), and in Peru (Aquino et al., 2022), both of which use the LiDAR change data as reference/ calibration (Figure 2 and Figure 5). For these analyses, the GNSS receivers were critical in ensuring that various UAV LiDAR datasets collected across multiple were correctly aligned, allowing the change in canopy cover to be measured without any meaningful error (i.e. differences are in single digit cm, not in metres). Additional work will focus on the mapping of aboveground carbon stocks, and its changes over time by combining the UAV and TLS data, which again required the UAV data to be accurately aligned both in space, and in time to allow these datasets to be merged, and individual trees to be matched between time points. All published manuscripts have been tagged in PURE the University of Edinburgh’s Research Information System as using GEF equipment.
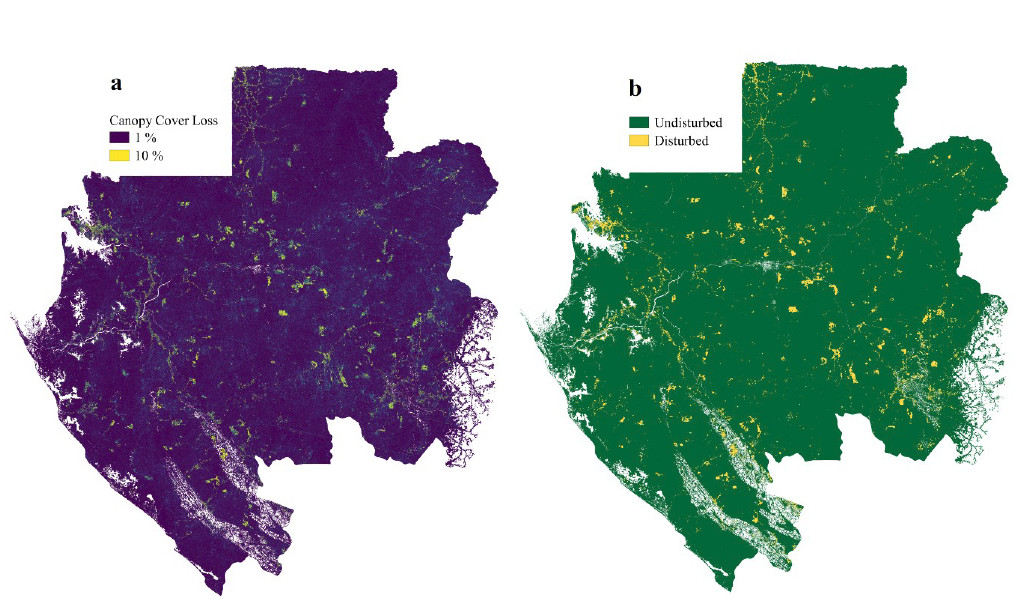
Data ArchivePermalink
All of D-GNSS raw data are stored in the NERC GEF facility hard drives in Edinburgh. In addition, geo-referenced and registered UAV-LiDAR data in the .las format, and Digital Area Photographs are stored on the Mitchard Research Group Datastore, which is located on the University of Edinburgh servers and automatically backed-up. The raw data will also be deposited on the Edinburgh DataShare repository (https://datashare.is.ed.ac.uk/) where it will be freely available for sharing. All the raw LiDAR, RGB imagery, and GNSS data will be stored on the NERC CEDA.
ConclusionsPermalink
As with all of our previous campaigns, the work under Loan 1136 was deemed a success and allowed us to complete the final part of the data collection under the FODEX project. The GNSS data were critical to this achievement, allowing us to perform the necessary PPK corrections on the UAV flight trajectories, and collect valuable GCPs, which aided the construction of the 3D point clouds. Our experience has taught us that care should always be taken to ensure that the base station is placed in a secure and open location, away from human interference, and that GCPs should be included in all measurement campaigns, even if just to act as validation points. The data collected as part of these Loans (1109 & 1124) has already led to five publications, with another three to be submitted by February 2024.
References / Publications from previous Loans (1109 & 1124)Permalink
Aquino, C., Mitchard, E.T.A., McNicol, I.M., Carstairs, H., Burt, A., Puma Vilca, B.L., Obiang Ebanéga, M., Modinga Dikongo, A., Dassi, C., Mayta, S., Tamayo, M., Grijalba, P., Miranda, F., Disney, M., 2022. Reliably mapping low-intensity forest disturbance using satellite radar data. Front. For. Glob. Change 5, 1018762. https://doi.org/10.3389/ffgc.2022.1018762
Carstairs, H., Mitchard, E.T.A., McNicol, I., Aquino, C., Burt, A., Ebanega, M.O., Dikongo, A.M., Bueso-Bello, J.L., Disney, M., 2022a. An Effective Method for InSAR Mapping of Tropical Forest Degradation in Hilly Areas. Remote Sensing 14, 124. https://doi.org/10.3390/rs14030452
Carstairs, H., Mitchard, E.T.A., McNicol, I., Aquino, C., Chezeaux, E., Ebanega, M.O., Dikongo, A.M., Disney, M., 2022b. Sentinel-1 Shadows Used to Quantify Canopy Loss from Selective Logging in Gabon. Remote Sensing 14, 4233. https://doi.org/10.3390/rs14174233
Davenport, I.J., McNicol, I., Mitchard, E.T., Dargie, G., Suspense, I., Milongo, B., Bocko, Y.E., Hawthorne, D., Lawson, I., Baird, A.J., Page, S., Lewis, S.L., 2020. First evidence of peat domes in the congo basin using LiDAR from a fixed-wing drone. Remote Sensing 12. https://doi.org/10.3390/rs12142196
McNicol, I.M., Mitchard, E.T.A., Aquino, C., Burt, A., Carstairs, H., Dassi, C., Modinga Dikongo, A., Disney, M.I., 2021. To What Extent Can UAV Photogrammetry Replicate UAV LiDAR to Determine Forest Structure? A Test in Two Contrasting Tropical Forests. Journal of Geophysical Research: Biogeosciences 126, 117. https://doi.org/10.1029/2021JG006586
Tables and FiguresPermalink
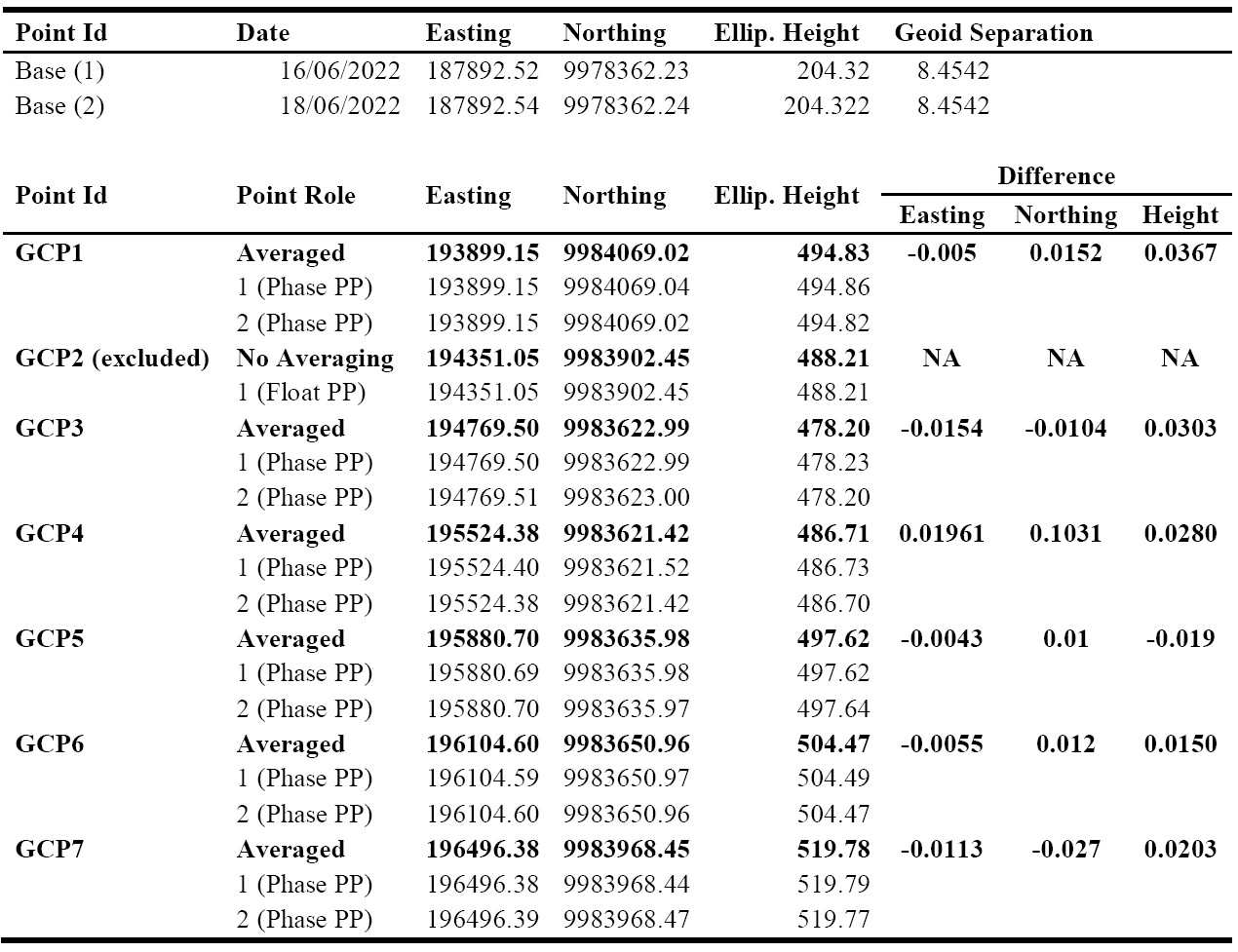
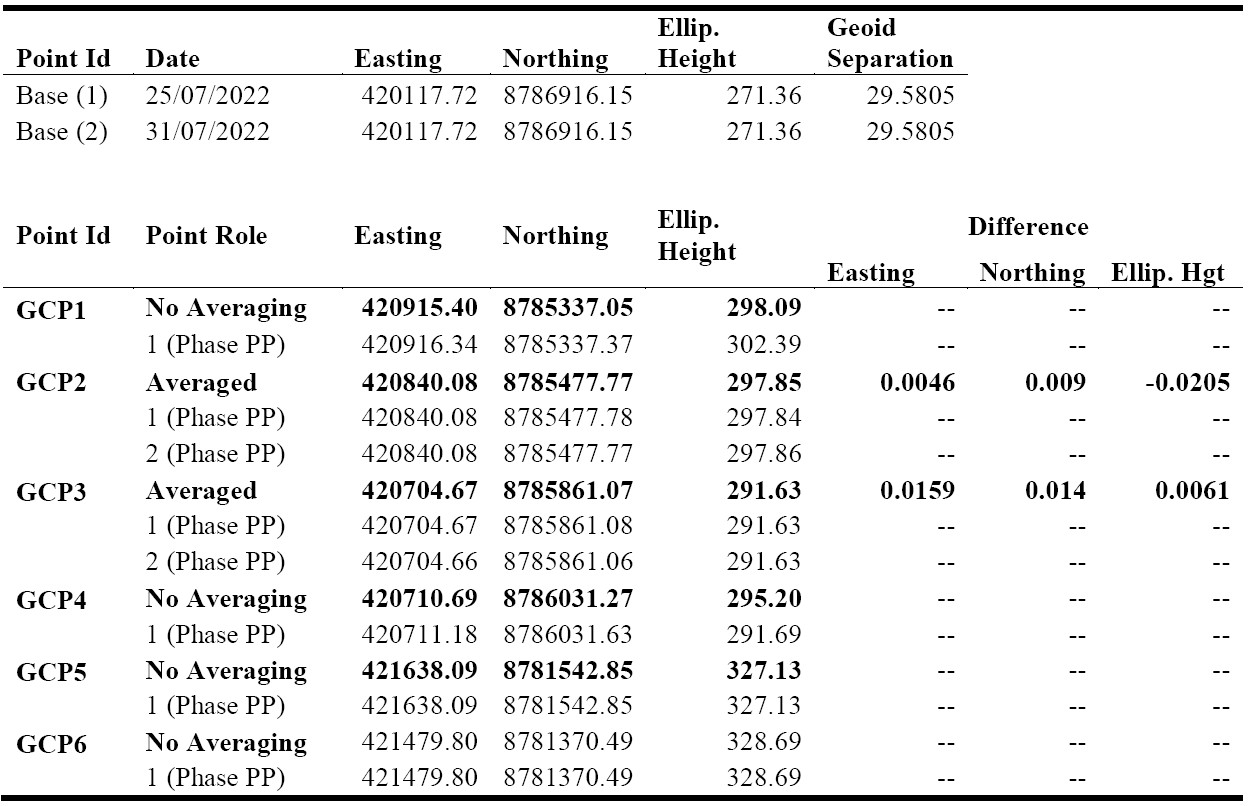